Inducing more reviews may not help the market and other thoughts on the difficulty of improving reputation systems
This blog is written by Andrey Fradkin, with special thanks to David Holtz.
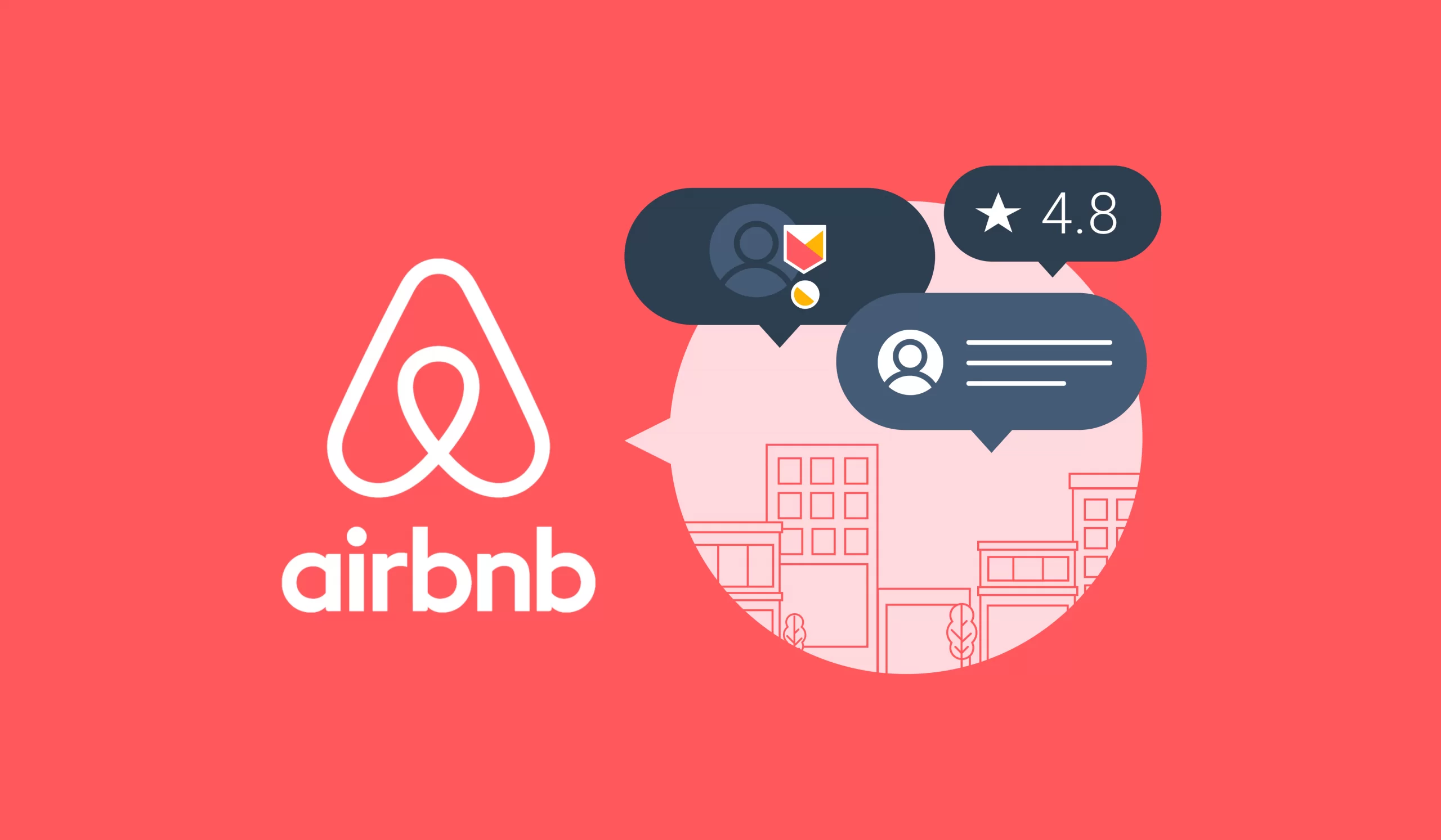
Reviews are everywhere on digital platforms, yet it is common knowledge that review systems are flawed. Not all users contribute reviews and those who do may have unusual experiences or preferences. In the course of writing a review, the lack of an objective standard for mapping experiences to ratings leads to inconsistencies amongst reviewers. Reviewers may also leave out relevant details to appear amicable or may exaggerate negative experiences as a form of retaliation. Despite these issues being widely recognized and discussed in the media, it seems review systems have barely improved over the past two decades. In this blog post, I will delve into the challenges of measuring reputation system improvements by examining a recent paper by David Holtz and myself.
Before discussing our results, it’s useful to consider the purpose of review systems. Review systems were introduced in the earliest digital marketplaces such as eBay to solve the problem of transacting with anonymous strangers on the internet, who could misrepresent their product or fail to deliver it altogether.[1] Reputation systems incentivized sellers to act honestly while providing buyers with the information needed to avoid potentially low-quality sellers. This created a virtuous cycle where more buyers began to trust eBay, while sellers joined eBay for access to a larger market.
While the success of eBay’s reputation system demonstrates the utility of reputation systems for most marketplaces, it doesn’t provide a blueprint for designing the best system. Platform designers must choose how to solicit reviews and how to display information from reviews. Startup platforms begin with a reputation system that is chosen by early employees based on intuition. As platforms grow, they have the opportunity to use data and experiments to improve these reputation systems. Yet the seemingly unchanging design of reputation systems suggests that this opportunity is not being seized as much as it should be.[2]
One reason for the lack of large changes to reputation systems is the lack of clarity about what signals are indicative of a better reputation system. Data scientists and researchers are often tempted to focus on immediate outcomes that are easier to measure. For example, policies such as reminders, nudges, and incentives can be shown to improve the quantity of reviews.[3] The effects of these policies on reviews are easy to evaluate because reviews come shortly after interventions, and it’s easy to map treatment assignment of potential reviewers to review behavior.
It is more difficult to measure how shifts in reviews translate to improved outcomes for the market. In a forthcoming paper in Marketing Science, David Holtz and I take an initial step in mapping review policy to market outcomes using a large-scale field experiment on Airbnb. The experiment was assigned at an Airbnb listing level for listings which did not yet have reviews. For treatment listings, buyers were offered a $25 coupon post-transaction to review. For the control listings, buyers were not offered any incentive to review after a transaction. The incentives increased review rates and induced more negative reviews (as measured through star ratings and text).
Our primary insight involves examining the impact of these reviews on the quantity and quality of matches for listings. To do so, we connect a listing’s initial treatment (whether a guest to the listing is offered a coupon to review) to its subsequent outcomes. Specifically, we track the listing outcomes for a year following the treatment assignment. This enables us to assess the effects of incentived reviews on the number of transactions and match quality indicators, such as subsequent reviews and complaints. We also provide a measure of match quality based on the subsequent experiences of guests who engaged in transactions with listings from the experiment. Note that this measurement exercise involves merging data up to two years of data after the experiment concluded.
We find that while the treatment induced more reviews, these reviews failed to improve listing outcomes both in terms of nights booked or in terms of match quality. In fact, we find that measures of match quality actually fell due to the treatment. Our key theory for the fall in match quality, which we support with additional analysis, is that the induced reviews were actually less correlated with quality than non-incentivized reviews. We find these results even though the induced reviews were more negative than non-incentivized reviews, which may naively suggest that they are more accurate.
Our results show that it is insufficient to only look at the quantity and valence of reviews in order to evaluate whether a reputation system design change improves market outcomes. Interestingly, this is our second failure to find benefits from a reputation system change on Airbnb. In a recently published paper, David Holtz, Elena Grewal, and I found that making the reputation system simultaneous reduced reciprocity and increased review rates. Yet, even with large samples, we failed to detect effects of this change on market outcomes.
Our findings, while specific to certain reputation system changes and the context of Airbnb, offer valuable insights into reputation system design more broadly. Had we solely focused on the effects of these changes on reviews, we might have erroneously concluded that these alterations significantly benefited the platform. Other platforms should also adopt this long-term analysis of transaction volume and match quality when experimenting with reputation system design.[4]
Circling back to the motivation of this post, I propose that a lack of innovation in reputation systems is, at least in part, due to the absence of a robust framework for evaluating changes in reputation system design. It is hard to advocate for resources in an organization when data scientists and product managers might struggle to assess the key performance indicators of a product change. It is also hard to advocate for longer-lived experiments and long-run analyses when product development cycles are often much shorter than a year.
What can data scientists and managers do to alleviate these constraints to improving and analyzing reputation systems? An obvious suggestion is to advocate for longer and more ambitious reputation system experiments. Additionally, it can be worthwhile to reserve time to re-analyze old experiments using a longer data horizon. Such re-analysis can yield surprising insights that will have implications for future design decisions. Statistical techniques such as surrogate analysis can also be used to learn about the longer-run effects of reputation system policies.
To summarize, by incorporating a comprehensive perspective that accounts for transaction volume and match quality, platforms can make better-informed decisions and design more effective reputation systems that genuinely benefit users and improve overall market outcomes.
This blog is based on Andrey’s research published in Management Science, which is included in the Platform Papers references dashboard:
Fradkin, A., & Holtz, D. (2023). Do incentives to review help the market? Evidence from a field experiment on Airbnb. Marketing Science.
Platform Paper Higlights
Let’s add a new feature to the blog (tell me how you like it, please). Here are some recently published papers on platform competition that stood out to me:
Sticking with the theme of reviews, a Journal of Consumer Research study by Rifkin, Kirk and Corus flips the perspective and ask what happens when it are consumers who are the ones being reviewed. The authors find that negative reviews of consumers (by sellers) on sharing economy platforms can harm the further diffusion of the platform. The negative effect can be attenuated by making reviews private (vs. public) and providing opportunities for justice restoration (e.g., response, revenge, dispute).
Andrey Fradkin is having a productive time! Another one of his papers together with co-authors Farranato and Fong recently got published in Management Science. The timely paper looks at the impact of horizontal mergers in the presence of network effects. They specifically study the merger of two US pet-sitting platforms, Rover and Dog Vaycay. When consumers have heterogeneous preferences for such services, the authors find, an increase in network effects following a merger can actually be offset by a loss in differentiation.
While platforms can have many benefits to both suppliers and buyers, one thing they often lack is a place of belonging or the feeling of being part of a team. A paper by Ai and co-authors published in Management Science studies what happens when drivers on a ride-sharing platform are randomly allocated into (virtual) teams. Compared with drivers in the control condition, treated drivers work longer hours and earn 12% higher revenue during the experiment. The effect, however, waned two weeks after the experiment.
In another study on ride-hailing platforms published in Management Science, Chung, Zhou and Ethiraj study the cross-platform competition effects from a platform’s governance policies. Specifically, when Lyft restricted drivers in New York City access to its platform due to tightned local regulations, Uber, too, saw its trip numbers reduced. The negative externality was also measured at times when access to Lyft was unrestricted. You could say that rising tides lift all boats, especially when their drivers can multihome!
These and 11 other papers were added to the Platform Papers references dashboard in the last month.
See you next month!
[1] Another concern in early marketplaces was that buyers would not pay, creating a role for seller reviews of buyers.
[2] Data and experimentation is often applied in soliciting reviews via notifications and emails and in using review information as a signal in ranking algorithms.
[3] Numerous studies have documented that these interventions do increase review rates and change the types of reviews which are submitted. These induced reviews are typically found to be more representative.
[4] There is also a separate literature that studies whether and how platforms tilt their reputation systems to promote transactions at the expense of match quality.